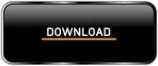
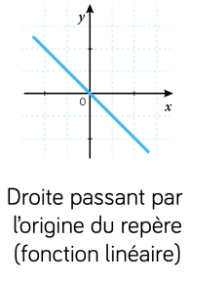
- Hyperplan vectoriel equation update#
- Hyperplan vectoriel equation windows 10#
- Hyperplan vectoriel equation Pc#
- Hyperplan vectoriel equation windows#
Hyperplan vectoriel equation windows#
It’s a strange problem, but there are ways to rectify this issue without having to reinstall Windows 10.
Hyperplan vectoriel equation update#
If you are seeing Black Bars in the middle, bottom or on the side of your screen or monitor, when playing games in Windows 10/8/7, then you need to update NVIDIA drivers, check your graphics settings, run the Troubleshooter, use windows full-screen mode, use native resolution, etc. Remove Black Bars from Games on Windows 11/10
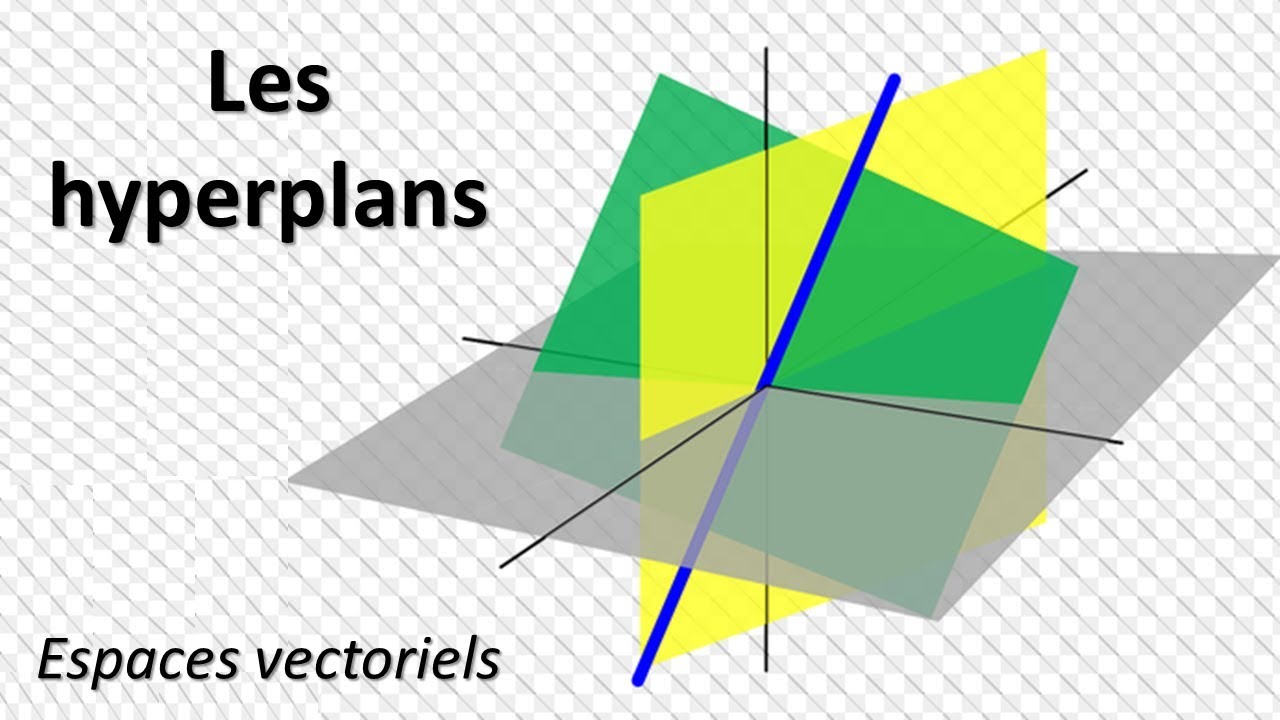
You see, from time to time, folks may come across black bars when playing their favorite video games.
Hyperplan vectoriel equation windows 10#
If you want to take advantage of what DirectX 12 has to offer, then Windows 10 is your only option.ĭespite all the good things Microsoft brings to the table, it doesn’t give a perfect experience. It’s the best place to play, and a lot of folks know this.
Hyperplan vectoriel equation Pc#
However, if we add new data points, the consequence of using various hyperplanes will be very different in terms of classifying new data point into the right group of class.If you want the best gaming experience, you’ll need a PC that is powered by Windows 10. As shown in the graph below, we can achieve exactly the same result using different hyperplanes (L1, L2, 元). To separate the two classes, there are so many possible options of hyperplanes that separate correctly. Imagine the labelled training set are two classes of data points (two dimensions): Alice and Cinderella. The hyperplane (line) is found through the maximum margin. i.e the maximum distance between data points of both classes.ĭon’t you think the definition and idea of SVM look a bit abstract? No worries, let me explain in details.

The objective of applying SVMs is to find the best line in two dimensions or the best hyperplane in more than two dimensions in order to help us separate our space into classes. Given a set of training examples, each marked as belonging to one or the other of two categories, an SVM training algorithm builds a model that assigns new examples to one category or the other, making it a non-probabilistic binary linear classifier. That’s why SVMs algorithm is important! What is Support Vector Machines (SVMs)? Therefore, the optimal decision boundary should be able to maximize the distance between the decision boundary and all instances. As we can see from the above graph, if a point is far from the decision boundary, we may be more confident in our predictions. Therefore, the decision boundary it picks may not be optimal. Logistic Regression doesn’t care whether the instances are close to the decision boundary. Hence, we’re much more confident about our prediction at A than at C
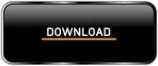